Efficient Reinforcement Learning with Relocatable Action Models
AAAI 2007
Pages
572-577
Resource Type
Proceedings
Realistic domains for learning possess regularities that make it possible to generalize experience across related states. This paper explores an environment-modeling framework that represents transitions as state-independent outcomes that are common to all states that share the same type. We analyze a set of novel learning problems that arise in this framework, providing lower and upper bounds. We single out one particular variant of practical interest and provide an efficient algorithm and experimental results in both simulated and robotic environments.
Associated Faculty
Unique ID
TR-2007-00041
Publication Date
Image
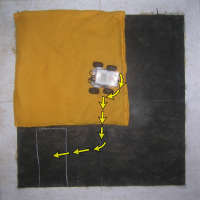