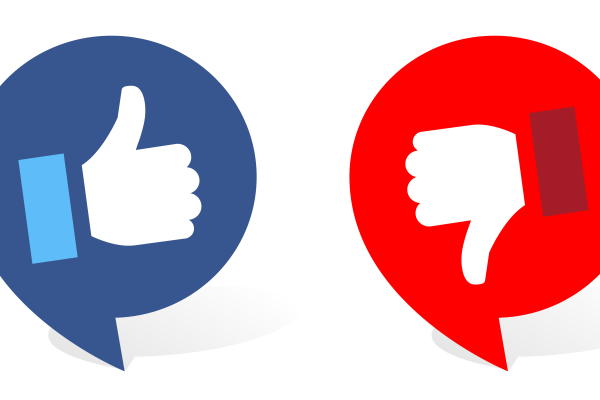
Research applies ‘Topic Modeling’ to Twitter for health policy-making
There is no doubt COVID-19 has brought out the best and the worst of us. One glance at COVID-related comments and discourse on Twitter illustrates that clearly.
So let’s say you’re a public health official in the middle of this pandemic crisis. How do you gauge common public sentiments in relation to the measures you’re employing or considering employing? You might want to go through social media to see what people are talking about in naturalistic settings. However, it could be exhausting and difficult to measure such large volumes and contrary opinions manually, one post at a time. Rolling out health policies is a delicate exercise, where public opinion matters greatly in terms of successful acceptance.
Natural Language Processing Tools Provide a Finger on the Pulse
In order to help with collecting and analyzing public opinion about COVID-19, Hyeju Jang—a postdoctoral fellow who is supported by a CIHR Health System Impact Fellowship, and Professor Giuseppe Carenini, her academic supervisor at UBC Computer Science—applied Natural Language Processing (NLP) techniques to social media data. The work was done in collaboration with experts in the BC Centre for Disease Control (BCCDC). The research is published in the Journal of Medical Internet Research (JMIR) entitled, “Tracking COVID-19 Discourse on Twitter in North America: Infodemiology Study Using Topic Modeling and Aspect-Based Sentiment Analysis.”
In the research, they analyzed COVID-19 related tweets using topic modeling and aspect-based sentiment analysis (ABSA). They compared the timelines of topics discussed on Twitter with the timing of interventions, and also investigated anti-Asian racism of North Americans by comparing timelines of sentiments toward the aspect words "Asians" and "Canadians".
Topic modeling identified 20 topics that were COVID related. The interpretation and timeline analysis showed that the trending topics are highly related to public health promotions and interventions such as physical distancing, border restrictions, handwashing, staying home, and face coverings.
After training the data using ABSA, they obtained 545 aspect terms like “vaccines,” “economy,” and “masks,” and 60 opinion terms such as “infectious” (negative) and “professional” (positive), which were used for inference of sentiments of the 20 key aspects selected by public health experts. The results showed negative sentiments related to the overall outbreak, misinformation and Asians, and positive sentiments related to physical distancing.
Analyses using natural language processing techniques with domain-expert involvement can produce very useful information for public health. This kind of information could help agencies to understand public concerns when designing policies for new interventions.
Jang explains how their techniques can also be applied broadly. “This methodology can help with COVID-19 vaccinations and how they’re rolled out for example,” she said. “But even beyond that, sentiment-analysis can potentially be applied to many other similar datasets.”
Carenini says he believes, “There should be solid investment in Natural Language Processing Research in health care similar to what is happening in e-commerce, finance, and media. Also, public health organizations that depend upon public sentiment would benefit from hiring people to use the new technologies and learn from them.”
An excerpt from the publication in JMIR:
“Our analyses demonstrated that Twitter conversations about COVID-19 are highly aligned with public health interventions. In our study, public health experts were actively involved in the computational process as well as interpretation of the results. The human-in-the-loop ABSA allowed manually editing aspect and opinion lexicons, and as a result, our analysis showed sentiments toward the aspects public health experts were interested in by leveraging the domain-specific lexicons. Our results suggest that monitoring Twitter user’s reactions about COVID-19–related aspects can be beneficial for public health policy makers.”
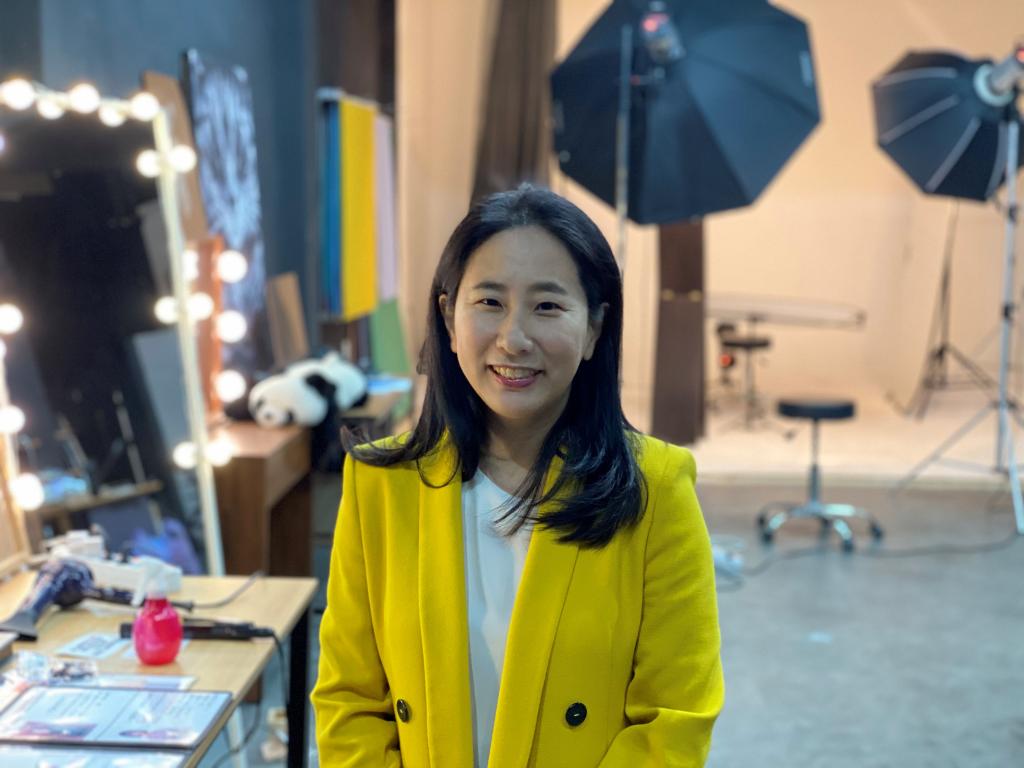
UBC Computer Science
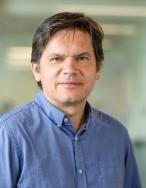