Prestigious computer graphics award acknowledges Dr. van de Panne’s career contributions
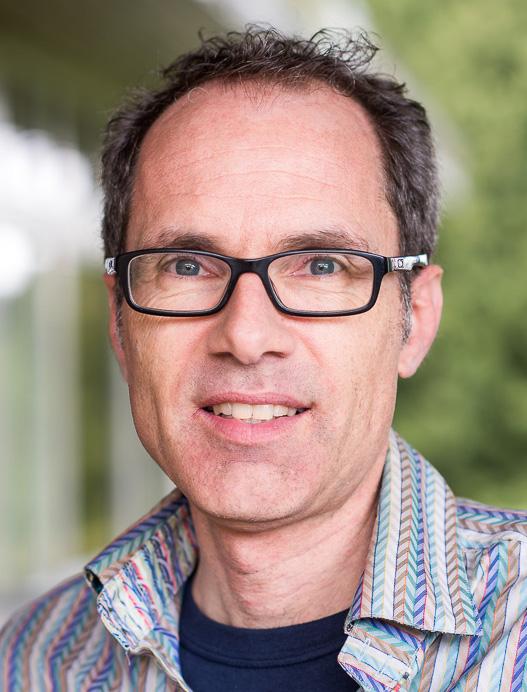
Professor Michiel van de Panne of UBC Computer Science has received the 2022 ACM SIGGRAPH (Special Interest Group on Computer Graphics and Interactive Techniques) Achievement Award and been named to the SIGGRAPH Academy. This prestigious award is given annually to one individual for making sustained and significant contributions to a specific area of computer graphics.
Dr. van de Panne is a Professor of Computer Science at the University of British Columbia, before which he held faculty positions at the University of Toronto.
He has been prolific with his research over the years, publishing more than 100 papers in the field. Dr. van de Panne’s focus is on modeling agile human movement using physically-based simulations, with applications to computer animation and robotics. He combines physics-based simulation, control theory and machine learning (especially reinforcement learning) to generate robust and reactive models of human locomotion and a growing variety of other motor skills.
“Michiel has advanced the field by suggesting new directions that have resulted in large steps forward,” the SIGGRAPH citation states. It also says, “His projects often start with a new ambitious task in computer graphics that challenges existing solutions, and finishes with an insightful piece of new knowledge that extends the state-of-the-art in machine learning.” Michiel’s work reverberates throughout the computer graphics and robotics communities.
In winning the award, Michiel is appreciative of the recognition, stating, “It feels wonderful to be recognized by the community after working towards the creation of skilled simulated characters for 30+ years. I am proud to have been one of the people leading the charge for this important area in computer graphics. And I’m particularly proud of my many amazing graduate students and collaborators that really made it all happen. This kind of research is entirely a team sport.”
Learning to move
Learning new movement skills that may require agility, precision, and rapid adaptation is no easy task, as becomes obvious when we try to learn a new sport, for example. How can we download a “skill”? A longstanding goal of Michiel’s work is to create physics-based digital characters that have many, or most, of the kinds of movement skills that we all have as humans.
Getting the physics right is not the hard part, as the simulation of passive ‘rag doll’ motions has long been used to generate falling-type motions in games and simulations. Rather, the real challenge is the unknown computations involved in “motor control.” That is, learning to use perception and control to actively drive simulated muscles to achieve desired outcomes. “Ultimately, the control of movement can be seen as a kind of optimization problem,” said Michiel. “And over the years we’ve been able to do ever better at solving these problems to generate human-like movement skills. In the past half-dozen years, we’ve been able to make particularly large steps forward using machine learning methods, specifically reinforcement learning using neural networks.”
Putting robots to the test
Michiel said robotics has also been a great way to provide real-world validation of skills learned in simulation. “We have collaborated with one of the world's leading robotics companies, Agility Robotics, and its co-founders at Oregon State University on how to make our learning methods work for their large bipedal robot, Cassie. Our collaboration was one of the first to successfully use deep reinforcement-learning methods for a large robot, for robust and agile locomotion.”
An exciting part for Michiel is how the line between computer graphics and the real world has continued to blur. “With digital twins, we can model and simulate phenomena that can then be applied into the real world, and then also bring real-world scenarios into a simulated world for experimentation.”
Some of Michiel’s more ground-breaking papers over the years include:
2007 SIMBICON: Simple Biped Locomotion Control
2010 Generalized Biped Walking Control
2017 DeepLoco: Dynamic Locomotion Skills Using Hierarchical Deep Reinforcement Learning
2018 DeepMimic: Example-Guided Deep Reinforcement Learning of Physics-Based Character Skills
Michiel will be presented with his Achievement Award at the 2022 SIGGRAPH Conference in Vancouver, being held August 8-11 this year. He also has three papers that have been accepted for the conference. Read more about these papers, and the three other accepted papers from UBC Computer Science researchers.
More about SIGGRAPH
More about Michiel van de Panne