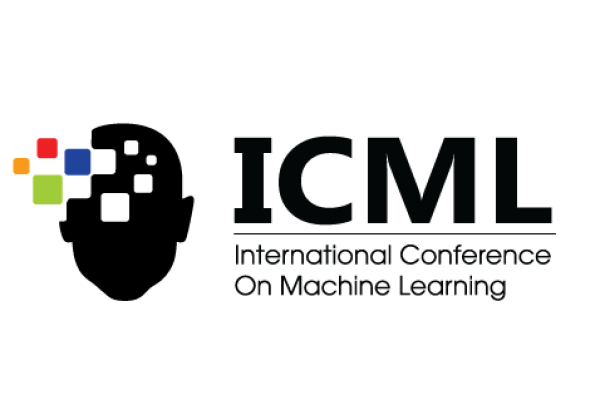
ICML Conference accepts six UBC Computer Science papers
Deep Learning. Deep research. Deep thinking.
In the world of machine learning, computer science researchers go to great lengths to further the abilities and mechanics of this rapidly flourishing discipline. The UBC Computer Science (CS) department is definitely no exception.
An impressive half dozen papers from the department have been accepted for the 37th Annual International Conference on Machine Learning (ICML), to be held virtually July 12th - 18th, 2020.
ICML is the leading international academic conference in machine learning. The conference is globally renowned for presenting and publishing cutting-edge research on all aspects of machine learning used in areas like artificial intelligence, statistics and data science, machine vision, computational biology, speech recognition, and robotics.
ICML is one of the fastest growing artificial intelligence conferences in the world. It’s no wonder, considering the global machine learning market is projected to grow from $7.3B in 2020 to $30.6B in 2024*, attaining a Compound Annual Growth Rate (CAGR) of 43%.
One of the paper's authors, UBC CS researcher Nick Harvey says, “My group’s paper is about Mirror Descent which is a fundamental algorithm used in Machine Learning. After working on this concept for over two years, we have now articulated how this fundamental algorithm works and can be analyzed. He continues, "Having six papers accepted at the conference from several different research groups, shows that UBC has a thriving presence in the machine learning community across a range of topics."
The papers accepted from UBC Computer Science are as follows:
All in the Exponential Family: Bregman Duality in Thermodynamic Variational Inference
Rob Brekelmans (USC Information Sciences Institute)*; Vaden W Masrani (University of British Columbia); Frank Wood (University of British Columbia); Greg Ver Steeg (USC Information Sciences Institute); Aram Galstyan (USC Information Sciences Institute)
Fiduciary Bandits
Gal Bahar (Technion – Israel Institute of Technology); Omer Ben-Porat (Technion – Israel Institute of Technology)*; Kevin Leyton-Brown (University of British Columbia); Moshe Tennenholtz (Technion – Israel Institute of Technology)
Generalized and Scalable Optimal Sparse Decision Trees
Jimmy Lin (University of British Columbia); Chudi Zhong (Duke University); Diane Hu (Duke University); Cynthia Rudin (Duke); Margo Seltzer (University of British Columbia)
Handling the Positive-Definite Constraint in the Bayesian Learning Rule
Wu Lin (University of British Columbia); Mark Schmidt (University of British Columbia); Mohammad Emtiyaz Khan (RIKEN)
Incidence Networks for Geometric Deep Learning
Marjan Albooyeh (University of British Columbia); Daniele Bertolini (N/A); Siamak Ravanbakhsh (McGill – Mila)
Online Mirror Descent and Dual Averaging: Keeping Pace in the Dynamic Case
Huang Fang (University of British Columbia); Nick Harvey (University of British Columbia); Victor Portella (University of British Columbia); Michael P Friedlander (University of British Columbia)
*Source: https://www.marketresearchfuture.com/reports/machine-learning-market-2494