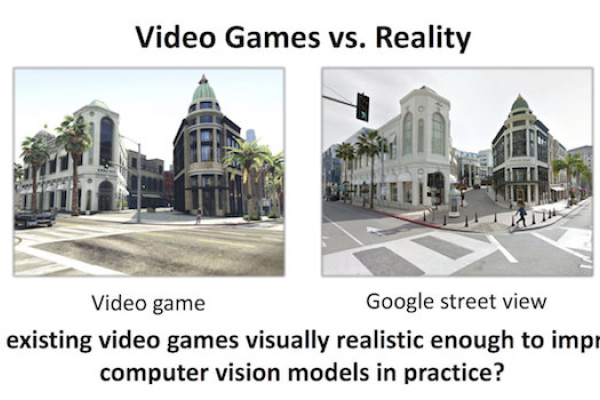
Video Games Help Teach Computer Vision Systems about the World
In recent years, deep learning has revolutionized how computer vision systems are developed, and made them more capable than ever before. These system work by learning from examples; in particular they require many real-world images that have been manually annotated to label the objects of interest. However the collection and annotation of these training images is
a slow and laborious process. An open question is whether computer graphics can by used as a convenient alternative method that directly generates as many annotated images as required for the learning. If the rendered training images are not sufficiently realistic, then the vision system will not do well on real images.
In research with his PhD supervisors, Jim Little and Mark Schmidt, Alireza Shafaei has found that training on images from the game performed just as well, and in some cases better than, training on available sets of real photos. These results suggest that large-scale datasets from video games can be used to help better train vision systems for self-driving cars, sports analysis, and any other visual domain where it would otherwise be difficult to obtain large sets of labeled images.
https://arxiv.org/abs/1608.01745
This work has also been picked up already by MIT Technology Review
and others:
https://www.technologyreview.com/2016/09/12/157605/self-driving-cars-can-learn-a-lot-by-playing-grand-theft-auto/