Researcher team makes great progress with Topic Segmentation within Natural Language Processing
PhD students Linzi Xing and Patrick Huber, along with Computer Science Professor Dr. Giuseppe Carenini have had their research paper, Improving Topic Segmentation by Injecting Discourse Dependencies published.
The paper has been accepted for the Computational Approaches to Discourse (CODI) 2022 conference taking place in October in South Korea.
The students work within the Natural Language Processing group, where Dr. Carenini is their supervisor.
Linzi explains the proposal, “Topic segmentation is an important task within the area of Natural Language Processing (NLP), with many real-world use-cases. As such, the topical structure of text is directly related to the underlying (often hidden), communicative goal of the author. Therefore, understanding semantic links and the context of a document plays an important role for the robust prediction of the document’s topical structure. In this work, we exploit the task of discourse dependency parsing (providing dependencies between parts of the text) to help separate input documents into topically coherent segments. We show that by injecting above-sentence structures with our graph modeling method, the segmentation performance improves substantially within and out-of-domain."
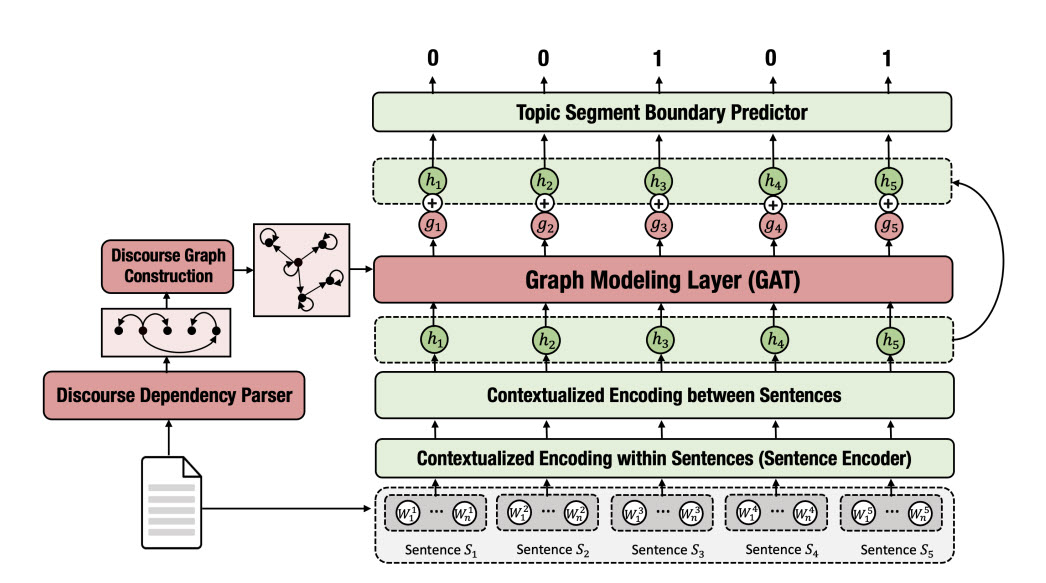