An optimal mix of solutions wins PhD grad an optimal prize
UBC PhD graduate Chris Liaw has won the Canadian Mathematical Society (CMS) Blair Spearman Doctoral Prize for his dissertation, titled Optimal Algorithms for Experts and Mixtures of Gaussians.
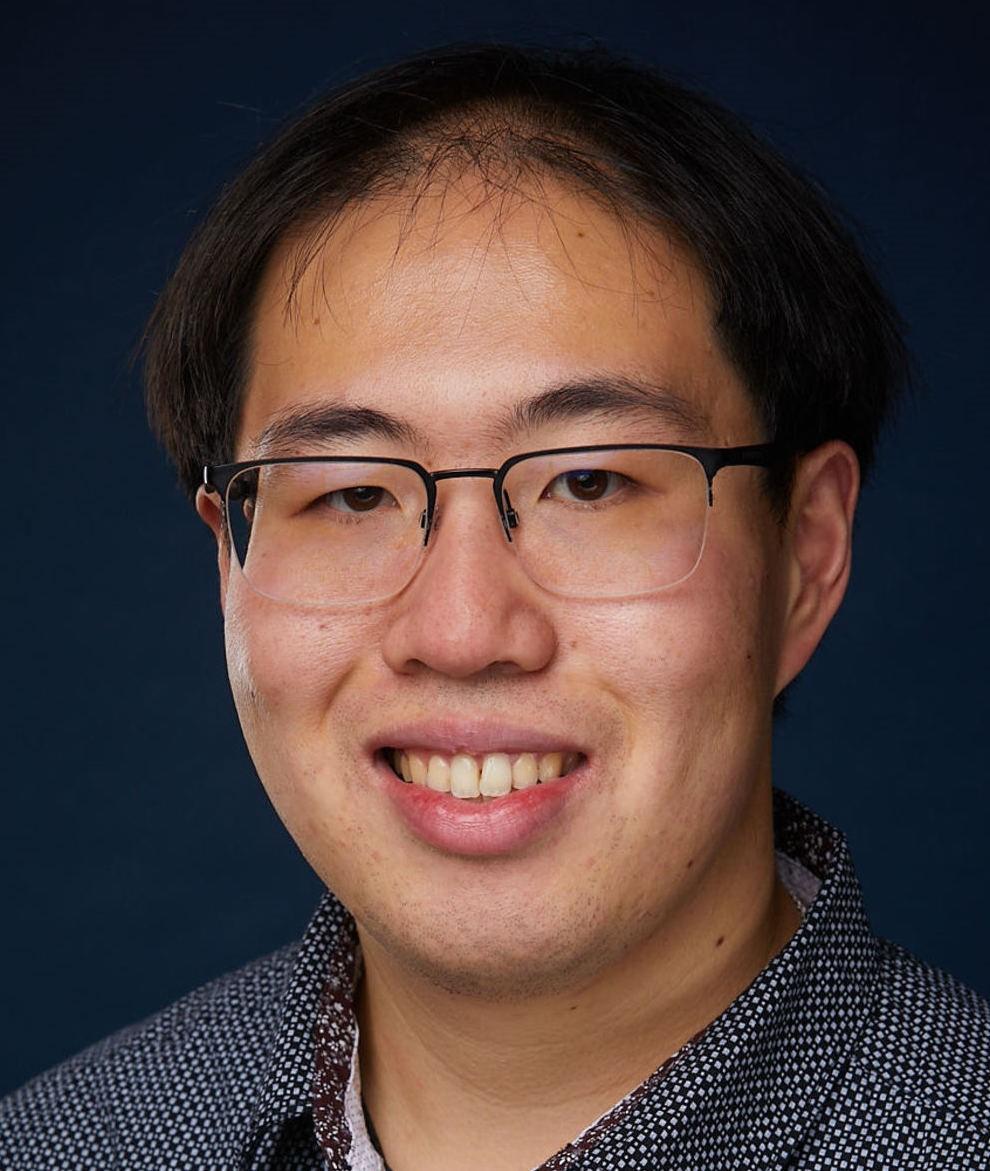
The award recognizes outstanding performance by a doctoral student from a Canadian university whose overall performance in graduate school is judged to be the most outstanding. Dr. Liaw, who is currently a postdoc in the Theory Group at the University of Toronto, will return to Vancouver to receive his award at the CMS Winter meeting.
A recent media release published by the CMS explains Chris’ work, which focuses on two questions at the intersection of theoretical computer science and machine learning:
“Christopher Liaw is an outstanding researcher whose work has contributed fundamentally to mathematical foundations of machine learning. The first problem he set out to solve is identifying the sample complexity of learning mixtures of Gaussians — a long-standing open problem, with previous solutions requiring extra assumptions.
Gaussian mixture models are probabilistic models for representing normally distributed subpopulations within an overall population. Mixture models in general don't require knowing which subpopulation a data point belongs to, allowing the model to ‘learn’ the subpopulations automatically.
Working together with several collaborators (Hassan Ashtiani, Shai Ben-David, Nick Harvey, Abbas Mehrabian, and Yaniv Plan), Dr. Liaw gave a precise characterization with minimal assumptions. Moreover, this work developed a new tool for distribution learning, which has since been applied to give the sample complexity for learning other classes of distributions. This resulted in a Best Paper award at NeurIPS 2018, an extraordinary distinction.”
The release went on to explain the second part of his dissertation:
“Christopher Liaw considers online predictions with expert advice, which is a classic model in learning theory. The problem is to find an optimal algorithm to choose a probability distribution over experts where at each day each expert receives a reward and the algorithm receives the expected reward under the chosen distribution. The goal is that, at all times, the total reward earned by the algorithm so far must nearly equal the maximum total reward of any expert by that time. It has been known for decades that there is an algorithm whose reward is only 𝑂(√𝑡 ln 𝑛) smaller than the best expert’s reward and this is optimal up to constants. The open question of finding the optimal constant has been posed as early as 1997. Liaw’s work (joint with Nick Harvey, Ed Perkins, and Sikander Randhawa) resolved this question exactly for n = 2.”
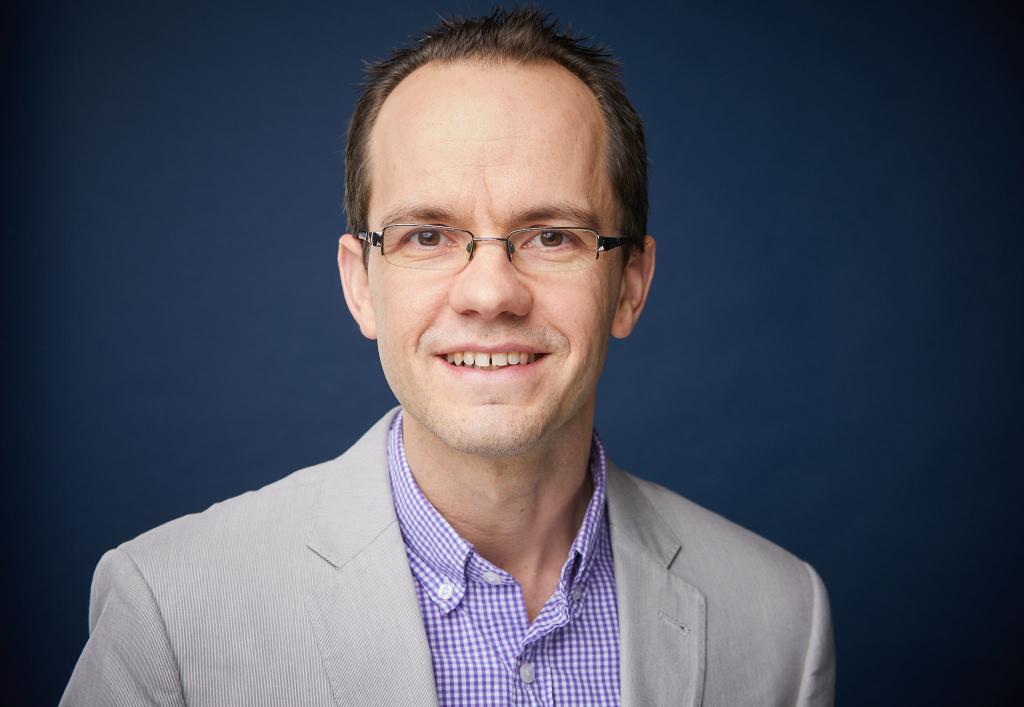
Chris was ecstatic to receive the prize and credits his supervisor Dr. Nick Harvey for much of his success. “I was nominated by my supervisor and he was instrumental in my winning the award. Nick, with his exceptional guidance in the projects he involved me in, as well as the extraordinary project team members, were all extremely helpful. I actually feel very fortunate so many things went right along the way, leading me to where I am today.”
Today, Chris’ research involves designing differentially private algorithms, which protect any personal from being discovered or leaked about each individual user.
As far as the future goes, Chris says, “Well, one step at a time for me, and I remain open to whatever possibilities await.”
We’re pretty sure those possibilities will be optimal, Chris.
About the Canadian Mathematical Society (CMS)
The CMS is the main national organization whose goal is to promote and advance the discovery, learning and application of mathematics. The Society’s activities cover the whole spectrum of mathematics including: scientific meetings, research publications, and the promotion of excellence in mathematics competitions that recognize outstanding student achievements.