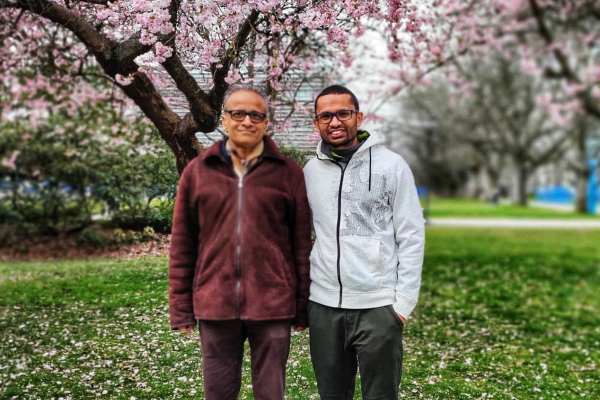
Paper explores diminishing bias and prejudice in online content
Like or agree with an opinion you read online? Great. You engage with that content and boom: you get MORE of that.
But will you then also receive an equal amount of opposing views in your feed? Not likely.
With the advent of AI and algorithms that are designed to give you more of what you show interest in, there is an inherent danger of resulting prejudice and bias. It is human nature that the more you are exposed to one opinion, the stronger your affiliation grows. In turn, this creates a ‘bubble’ of information. And then at the same time, the stronger your defiance grows against the opposing opinion.
These ‘bubbles’ of information are referred to as ‘filter bubbles.’ They are essentially filtering out opposing views. Naturally, filter bubbles can lead to one-sided policy decisions, social distrust, and other negative consequences. Within filter bubbles are ‘echo chambers.’ Echo chambers happen on social networks when users echo (share) information from a filter bubble because they strongly support the idea or opinion. In this case, the information is moving from a leader to a follower, and the follower is echoing it to their followers. And so on, and so on.
How can we correct the bias?
A computer science research team at the University of British Columbia has been working diligently to help solve the problem that arises when algorithms work too well in delivering opinions. Their novel approach has resulted in an accepted paper by the ACM SIGMOD Conference in Seattle this June.
The lead author of the paper “Mitigating Filter Bubbles Under a Competitive Diffusion Model” is UBC computer science alum, Dr. Prithu Banerjee. He was a PhD student at the time of the research, working under the supervision of Dr. Laks V.S. Lakshmanan.
He explained the problem, “If a piece of content is not challenging one’s viewpoint, one will stay there longer, and engage more. For business reasons like keeping users engaged longer and more frequently, large social networks want to encourage this. So the algorithms are designed to promote the aligning viewpoints. Another problem is that there is an inherent competition between two opposing viewpoints.” Prithu explained that this competition actually further divides and strengthens each opposing view. “The user, therefore, has a reduced likelihood of adopting the competitive viewpoint, if he has already been exposed more to the other viewpoint and connected with it.”
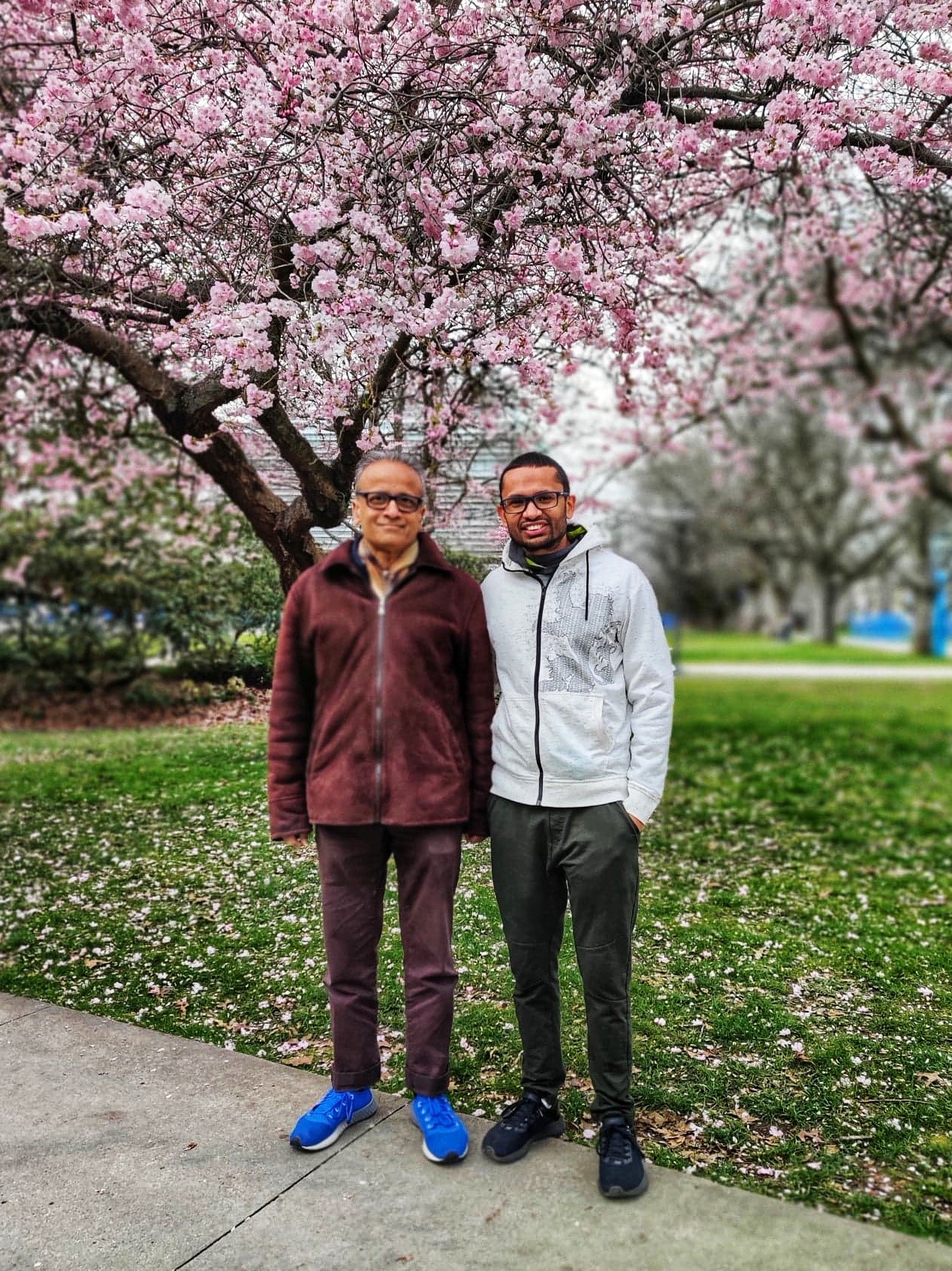
Drs. Banerjee and Lakshmanan also identified another factor: the ‘mitigation factor.’ Prior research assumed that a user begins with a clean slate of being exposed to both opinions on a particular subject at the same time and with the same level of exposure.
This in fact can not be true, he explains, because a user can only read one thing at a time. One piece of content has to be read first, before the other. “As a result, we will have to account for the bias that the first content has already introduced,” Dr. Banerjee said. This is the mitigation factor.
The UBC researchers have developed a novel way of mitigating said filter bubble problem. They have added a time delay between the propagation of the two opposing viewpoints so that the mitigation aspect can be captured.
They have also introduced a competition parameter. It predicts the probability of adopting a different viewpoint. Additionally, there are reward parameters they’ve introduced to help offset the problem of bias and ensure the user is equally exposed to both sides or viewpoints.
The results? Increased adoption of both opinions.
Banerjee said they used large-scale publicly available social networks like Orkut, to test how their methodologies performed and to study the polarities of opinion.
“You can’t really measure what a user is adopting,” he explained. “But you can use a proxy. The proxy we used is to measure adoption of what a user propagates to, or engages with, their followers, and if there is an increased engagement with both opinions.”
A gradual shift for social networks
Banerjee further explained that freedom of opinion and user engagement on both sides of the coin is not necessarily aligned with the business goals of large-scale social networks. “But, if we can give a network owner the ability to fine-tune their algorithms by employing these parameters, the hope is they will do something honourable, perhaps through a gradual implementation.”
He admits that sounds somewhat altruistic and wishful thinking, but also points out that in the long run, research studies have shown that over time, very strong biased opinions propagate a hostile environment. “And hostile environments ultimately drive people away. Social networks like Twitter don’t want to drive people away or lose users. They want to keep people around. That is their very livelihood.”
Banerjee suggests that by giving social media network owners the ability to refine their algorithms, and give people what they want without bias and prejudice, perhaps this would lead to a more balanced, less hostile environment, which benefits everyone.