|
Constrained Generative Modeling with Manually Bridged Diffusion Models
Saeid Naderiparizi, , ,
AAAI, 2025
(Oral Presentation)
Diffusion models on constrained domains. The constraints are specified by differentiable distance functions.
[arXiv]
|
|
Don't be so negative! Score-based Generative Modeling with Oracle-assisted Guidance
Saeid Naderiparizi, , , ,
ICML, 2024
Diffusion models under constraints specified by a given binary oracle
that determines whether a sampled data point is valid or not.
|
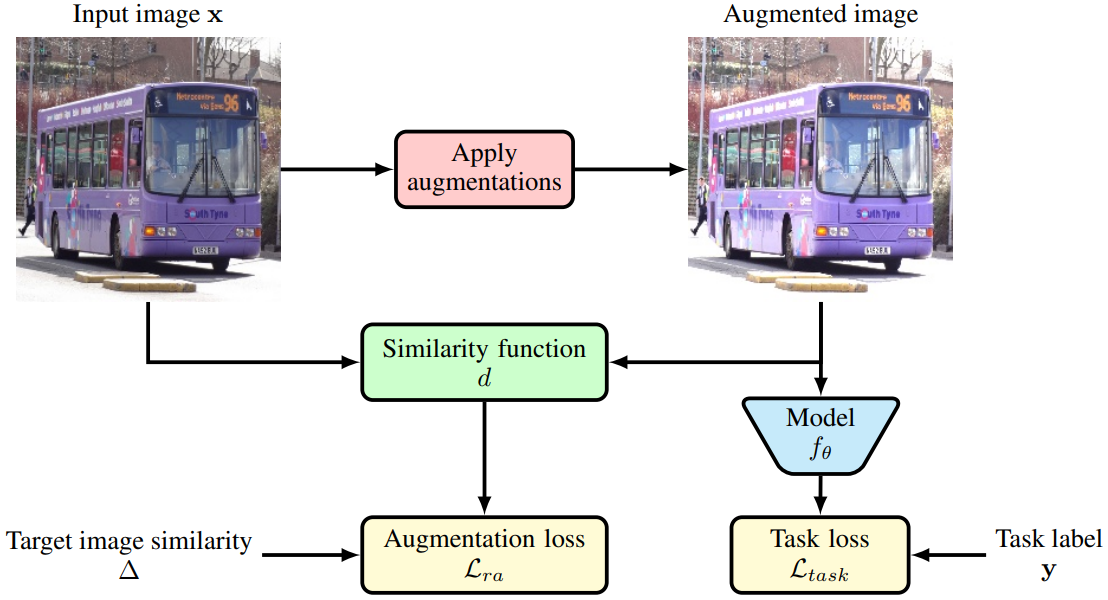 |
RangeAugment: Efficient Online Augmentation with
Range Learning
, Saeid Naderiparizi, ,
, ,
, ,
arXiv preprint, 2022
An automatic augmentation method that efficiently learns the range of augmentations together with
training the downstream model. On multiple large-scale computer vision tasks, it achieves competitive
performance with state-of-the-art augmentation methods, with 4-5 times fewer augmentation operations.
Work was done during my summer internship at Apple.
[Code (as a part of CVNets repository)]
|
|
Flexible Diffusion Modeling of Long Videos
, Saeid Naderiparizi, , ,
NeurIPS, 2022
Video generative models based on Denoising Diffusion Probabilistic Models (DDPMs). This model can generate videos of more than an hour in length.
[Project page]
[Code] [Videos] [arXiv]
|
|
Amortized Rejection Sampling in Universal Probabilistic Programming
Saeid Naderiparizi, , ,
,
, , ,
,
, , ,
AISTATS, 2022
(Oral Presentation)
[Top-9%]
Identifying and addressing the problems caused by rejection sampling loops in inference in
universal probabilistic programs.
[Code] [arXiv]
|
|
Conditional Image Generation by
Conditioning Variational Auto-Encoders
, Saeid Naderiparizi,
ICLR, 2022
Conditional image completion using amortized inference in image generative models, particularly,
variational auto-encoders.
We demonstrate that it leads to diverse and realistic generated images.
[Code] [arXiv]
|
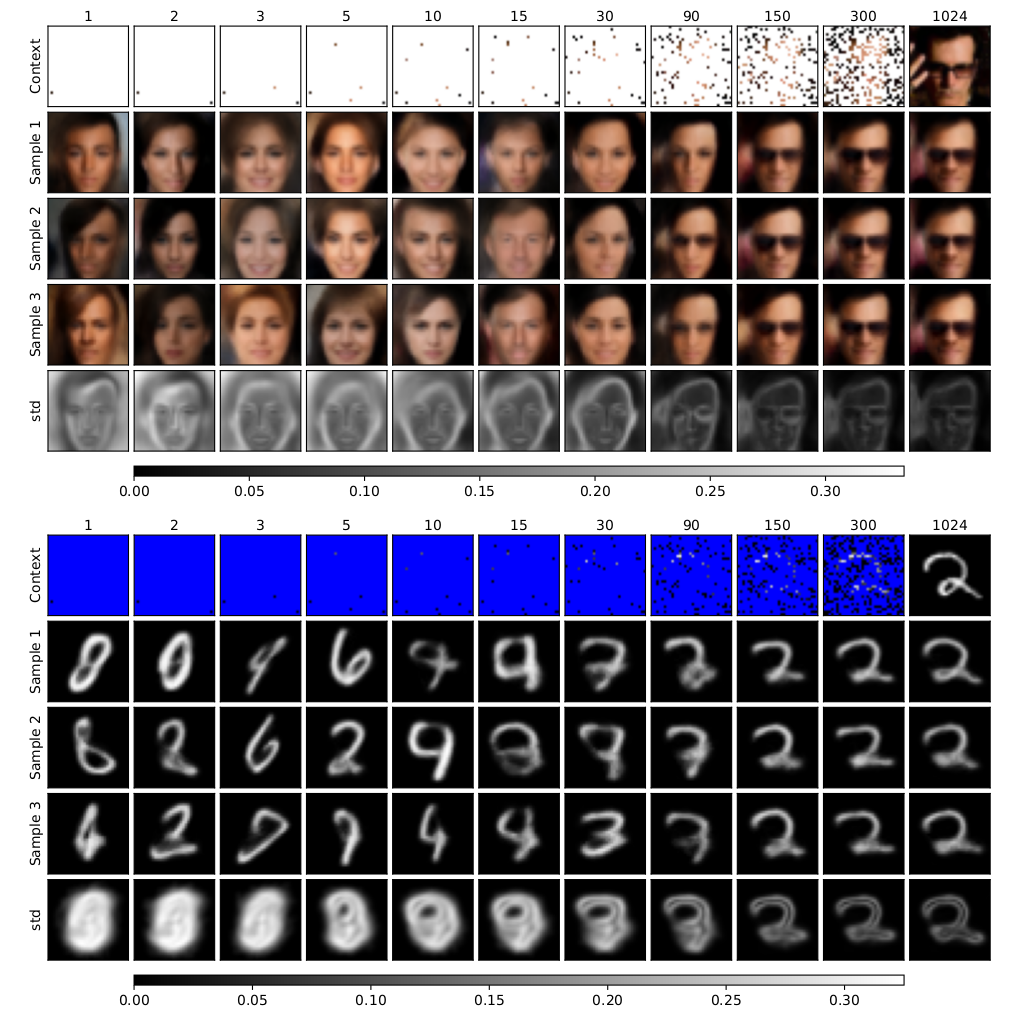 |
Uncertainty in Neural Processes
Saeid Naderiparizi, , ,
arXiv preprint, 2020
Achieving calibrated uncertainty in Neural Processes, especially in low data regime, via different
architectures and training objectives.
|
|
Planning as
Inference in Epidemiological Dynamics Models
, , Saeid Naderiparizi, , , ,
, ,
Frontiers in Artificial Intelligence, 2022
Automating parts of infectious disease-control policy-making via performing inference in existing
epidemiological dynamics models.
[Code] [Frontiers]
|
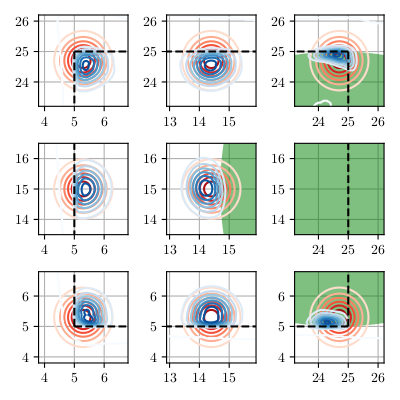 |
Coping With Simulators That Don't Always
Return
, Saeid Naderiparizi,
AISTATS, 2020
Model learning in models partially defined through "brittle" deterministic simulators.
A "brittle" simulator is one which would fail to produce results for invalid inputs that might occur
over the course of training.
An ODE solver not converging to the required tolerance in the allocated time or solid bodies
intersecting in robot simulators are examples of simulator failure.
[Code] [arXiv]
|
|
Efficient
Probabilistic Inference in the Quest for Physics Beyond the Standard Model
, , , ,
Saeid Naderiparizi, , ,
,
, ,
, ,
, ,
Neural Information Processing Systems (NeurIPS), 2019
A novel probabilistic programming framework that couples to the existing large-scale simulators via
a cross-platform protocol, allowing automatic inference in such simulators.
|
|
Etalumis: bringing
probabilistic programming to scientific simulators at scale
, , , ,
, , ,
Saeid Naderiparizi, ,
,
, ,
,
, , ,
Proceedings of the International Conference for High Performance Computing, Networking, Storage, and
Analysis, 2019
|
|