Research Interests
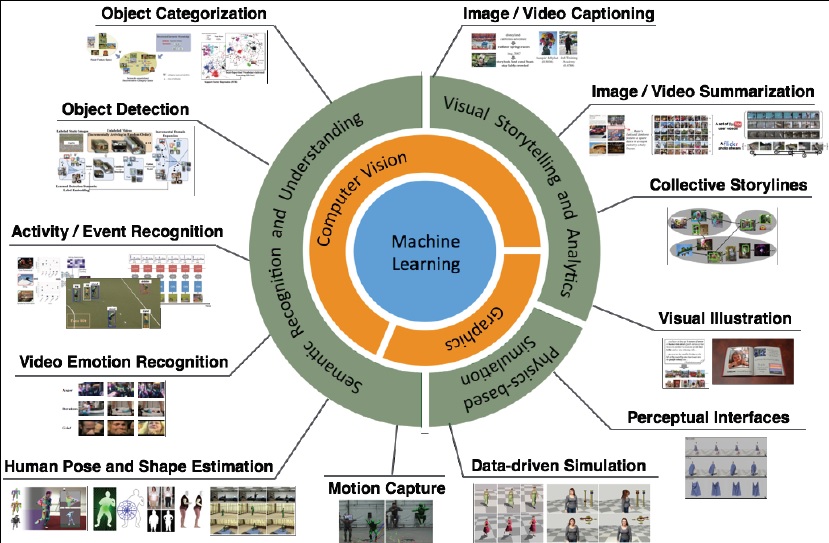
I am interested in problems of visual recognition and understanding, which include classical computer vision problems such as object categorization and detection, video segmentation, human pose and shape estimation, and video activity / event recognition, as well as more recent problems of video caption-ing, visual question answering, storytelling and analytics. These problems inherently demand novel machine learning and statistical methods to solve, as well as have the breadth of potential applications, that span curation of large visual media collection, human-robot interactions, and visual-powered analytics, to name a few.
Over the last 4-5 years the field has seen a great deal of growth, that, to a large extent, can be attributed to the success of deep learning architectures, such as Convolutional Neural Networks (CNN) and Recurrent (RNN) Neural Networks, and abundance of larges-scale annotated datasets needed to train recognition models of these forms. However, a number of significant challenges remain. My research focuses on some of these challenges, including: ability to effectively exploit contextual structure among elements of the scene to improve understanding; computational models that are able to scale without requiring large amounts of training data for each and every category being recognized; ability to effectively leverage and learn from unlabeled, weakly labeled, or partially labeled data; study of interplay between natural language and vision as means of extracting semantic knowledge that can improve semantic recognition; and ability to ascertain certain common sense knowledge directly from the visual domain.
I am also interested in problems in computer graphics. My research in this space focuses on approaches for human motion capture and modeling of complex physical phenomena, such as simulation of cloth and hair. Motion capture, a process of recording of articulated movement of a human actor, is a fundamental technology for animation in any virtual game or movie production. While it is possible to capture a subject accurately in a constrained laboratory or studio environment, the real challenge is doing so from relatively few markers and in an unencumbered space. In my research, I addressed these challenges by developing approaches that used non-traditional sensors (e.g., small cameras strapped to the body), combinations of sensors (e.g., retro-reflective markers and IMUs), and/or physics-based models of the human body as ways to regularize noisy and physically implausible estimates. My interest in physics-based modeling also extends to other complex phenomena, such as cloth and hair. For these problems, with the help of machine learning techniques, I have developed a class of data-driven simulation methods. Data-driven simulation methods learn proxy models that can approximate most of the physical behavior, at very low computational costs, allowing them to be applied in real-time applications or where, previously, computational costs were prohibitive.