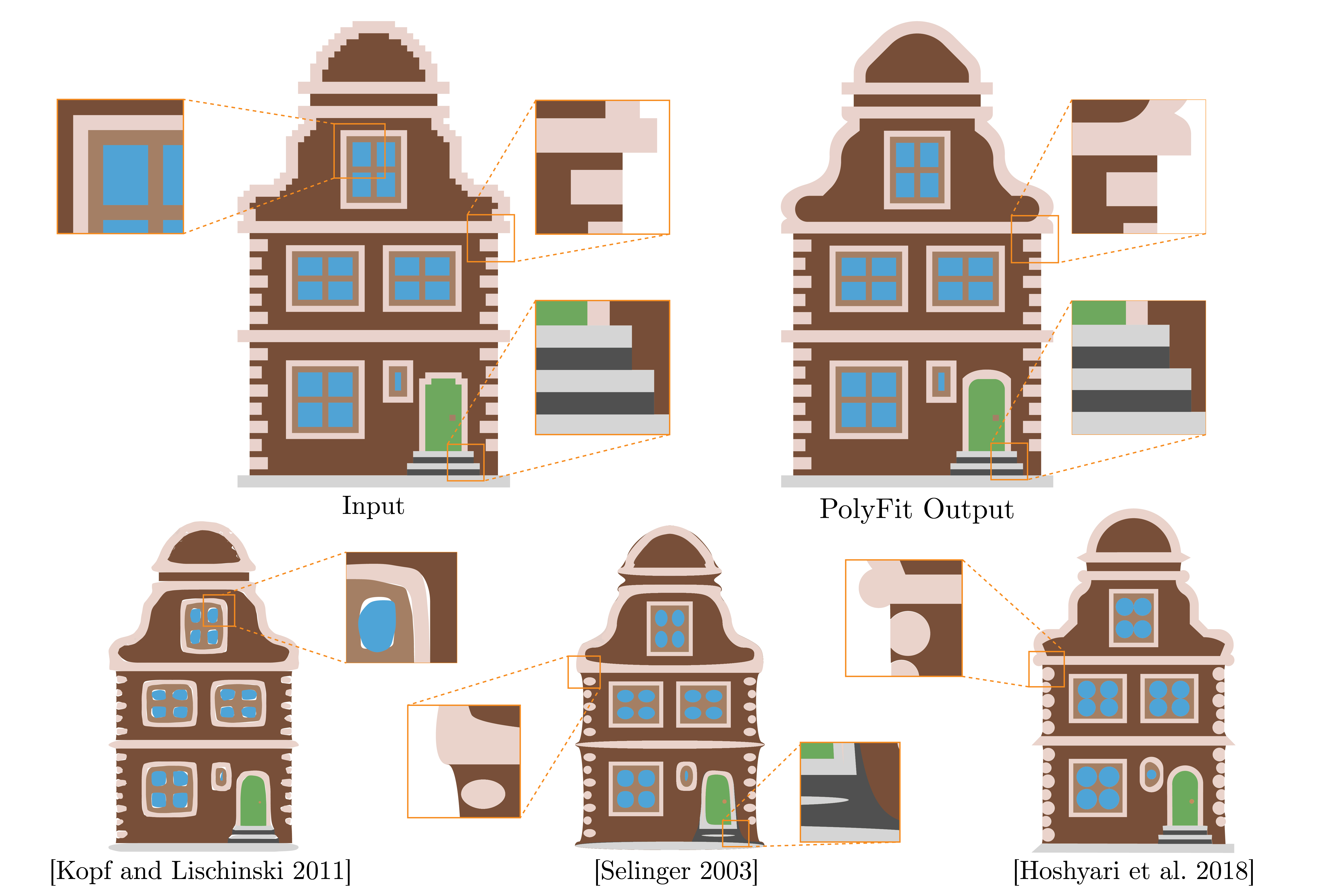
Raster clip-art images, which consist of distinctly colored regions separated by sharp boundaries typically allow for a clear mental vector interpretation. Vectorizing these images can facilitate compact lossless storage and enable numerous processing operations. Despite recent progress, existing vectorization methods that target this data frequently produce vectorizations that fail to meet viewer expectations. We present PolyFit, a new clip-art vectorization method that produces outputs well aligned with human preferences. Since segmentation of such inputs into regions had been addressed successfully, we focus on fitting piecewise smooth vector curves to the raster input region boundaries, a task prior methods are particularly prone to fail on. While perceptual studies suggest the criteria humans are likely to use during mental boundary vectorization, they provide no guidance as to the exact interaction between them; learning these interactions directly is problematic due to the large size of the solution space. To obtain the desired solution, we first approximate the raster region boundaries with coarse intermediate polygons leveraging a combination of perceptual cues with observations from studies of human preferences. We then use these intermediate polygons as auxiliary inputs for computing piecewise smooth vectorizations. We define a finite set of potential polygon to curve primitive maps, and learn the mapping from the polygons to their best fitting primitive configurations from human annotations, arriving at a compact set of local raster and polygon properties whose combinations reliably predict human-expected primitive choices. We obtain the final vectorization by fitting the computed primitive sequence to the raster data. Comparative user studies show that our method outperforms state-of-the-art approaches on a wide range of data; our results are preferred three times as often as those of the closest competitor across inputs with various resolutions. On low-resolution color data, this preference grows to a ratio of more than 15:1.
Source Code (Github)
Polygon Tracing Study (4 MB)
User Study (4 MB)
Training Data (1 MB)
More Results (9 MB)
@article{ dominici2020vectorization, author = {Alberto Dominici, Edoardo and Schertler, Nico and Griffin, Jonathan and Hoshyari, Shayan and Sigal, Leonid and Sheffer, Alla}, title = {PolyFit: Perception-aligned Vectorization of Raster Clip-art via Intermediate Polygonal Fitting}, journal = {ACM Transaction on Graphics}, year = {2020}, volume = {39}, number = {4}, doi = {10.1145/3386569.3392401}, publisher = {ACM}, address = {New York, NY, USA}, }
Note: some of the input images shown are copyrighted by third parties and used with their permission. See the paper for the list of third-party sources.